Financial institutions are moving beyond pilot projects to implement production-grade, explainable and cost-effective AI solutions that can meet operational and regulatory demands.
AI has evolved rapidly since fintech Arteria AI was founded in 2020, Amir Hajian, chief science officer, tells Bank Automation News in this episode of “The Buzz” podcast. The company provides banks with AI-powered digital documentation services.
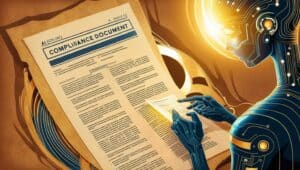
“2020 was a very simple year where AI was classification and extraction, and now we have all the glory of AI systems that can do things for you and with you,” Hajian says.
“We realized one day in 2021 that using language alone is not enough to solve [today’s] problems.” The company began using multimodal models that can not only read but search for visual cues in documents.
AI budgets and strategies vary widely among FIs, Hajian says. Therefore, Arteria’s approach involves reengineering large AI models to be smaller and more cost-effective, able to run in any environment without requiring massive computer resources. This allows smaller institutions to access advanced AI without extensive infrastructure.
Hajian, who joined Arteria AI in 2020, is also head of the fintech’s research arm, Arteria Cafe.
One of Arteria Cafe’s first developments since its creation in January is GraphiT — a tool for encoding graphs into text and optimizing large language model prompts for graph prediction tasks.
GraphiT enables graph-based analysis with minimal training data, ideal for compliance and financial services where data is limited and regulations shift quickly. The GraphiT solution operates at roughly one-tenth the cost of previously known methods, Hajian says.
Key uses include:
- Regulatory compliance;
- Anti-money laundering;
- Predictive modeling; and
- Low-data problem solving.
Arteria plans to roll out GraphiT at the ACM Web Conference 2025 in Sydney this month.
Listen to this episode of “The Buzz” podcast as Hajian discusses AI trends in financial services.
Subscribe to The Buzz Podcast on iTunes or Spotify, or download the episode.
The following is a transcript generated by AI technology that has been lightly edited but still contains errors.
Madeline Durrett 14:12:58
Hello and welcome to The Buzz bank automation news podcast. My name is Madeline deret, Senior Associate Editor at Bank automation news today. I’m joined by arteria cafe Chief Science Officer, Dr Amir. Heijn Amir, thanks so much for joining me today.
14:13:17
Thank you for having me
Madeline Durrett 14:13:20
so you have a background in astrophysics. How did you find yourself in the financial services sector, and how does your experience help you in your current role?
Speaker 1 14:13:32
It has been a great experience, as you know, as an astrophysicist, my job has been solving difficult problems, and when I was in academia, I was using the big data of the universe to answer questions about the universe itself and the past and the future of the universe using statistical and machine learning methods. Then I realized I could actually use the same techniques to solve problems in everyday life, and that’s how I left academia and I came to the industry, and interestingly, I have been using similar techniques, but on a different kind of data to solve problems. So I would say the most useful skill that I brought with myself to to this world has been solving difficult problems, and the ability to deal with a lot of unknown and and walking in the dark and figuring out what the actual problem is that we have to solve, and solving it, that’s really interesting.
Madeline Durrett 14:14:50
So arteria AI was founded in 2020 and how have client needs evolved since then? What are some new problems that you’ve noticed emerging? And how does arteria AI address these problems?
Speaker 1 14:15:07
So in 2020 when I joined arteria in the early days, the main focus of a lot of use cases where, in the we’re focused on just language in the documents, there is text. You want to find something in the text in a document, and then slowly, as our AI got better, because we were using AI to solve those problems, and as we got better and and the models got better, we realized one day in 2021 actually, that using language alone is not enough to solve those problems, so we started expanding. We started using multi modal models and and building models that can not only just read, but they can also see and look for visual cues in in the documents. And that opened up this whole new direction for for us and for our clients and their use cases, because then when we talk to them, they started imagining new kind of problems that you could solve with those so something happened in 2021 2022 where we went beyond just the language. And then in the in the past couple years, we have seen that that image of AI to be used only to to classify and to find information and to extract information. That’s actually only a small part of what we do for our clients. Today, we will talk more about this. Hopefully we have, we have gone to building compound AI systems that can actually do things for you and and can use the information that you have in your data, and can be your assistance to to help you make decisions and and deal with a lot of fast changing situations and and and give you what you need to know and help you make decisions and and take a few steps with you to make it much easier and much more reliable. And this, when you when you look back, I would say 2020. Was very simple year where AI was classification and extraction. And now we have all the. Glory of AI systems that can do things for you and with you.
Madeline Durrett 14:18:01
And how does arteria AI integrate with existing banking infrastructure to enhance compliance without requiring major system overhauls
Speaker 1 14:18:12
seamlessly so the there, there are two aspects to to to your question. One is the user experience aspect, where you have you want to integrate arteria into your existing systems, and what we have built at arteria is something that is highly configurable and personalizable, and you can, you can take it and it’s a no code system that you can configure it easily to connect to and integrate with Your existing systems. That’s that’s one part of it. The other aspect of it, which is more related to AI, is based on our experience we have seen that is really important for the AI models that you build to run in environments that do not have huge requirements for for compute. As you know, when you say, AI today, everyone starts thinking about thinking about massive GPU clusters and all the cost and requirements that you would need for for these systems to work. What we have done at arteria, and it has been crucial in our integration efforts, has been re engineering the AI models that we have to distill the knowledge in those big AI models into small AI models that would learn from from the teacher models and and these smaller models are fast, they are inexpensive to run, and they can run in any environment. And a lot, a lot of our clients are banks, and you know, banks have a lot of requirements around where they can run they where they can put their data and where they can run these models. With what we have built, you can seamlessly and easily integrate arterios ai into those systems without forcing the clients to move their data elsewhere or to send their data to somewhere that they are not comfortable with, and as a result, we have an AI that you can use in real time. It won’t break the bank, it’s accurate, it’s very flexible, and you can use it wherever you want, however you want. So
Madeline Durrett 14:20:59
would you say that your technology benefits like maybe community banks that are trying to compete with the innovation strategy of larger banks when we don’t have the resources for a large language model exactly
Speaker 1 14:21:12
and because what, what we have seen is you don’t, you don’t require all the knowledge that is captured in in those massive models. Once you know what you want to do, you distill your knowledge into smaller models and and then it enables you as a smaller bank or or a bank without all the infrastructure to be able to use AI, and is a huge step towards making AI accessible by our by everyone.
Madeline Durrett 14:21:49
Thank you, and I know arteria AI’s technology can help banks and banks adhere to compliance regulations. How do you ensure the accuracy and reliability of AI generated compliance documents and ensure that your models are fair? What’s your strategy for that?
Speaker 1 14:22:12
So these are machine learning models, and we as humans, as scientists, have had decades of experience dealing with machine learning based models that are statistical in nature. And you know, being statistical in nature means your models are guaranteed to be wrong X percent of time, and that X percent what we do is we fine tune the models to make sure that the. Number of times the models are wrong, we minimize it until it’s good enough for the business use case. And then there are standard practices that we have been using all through, which is a we make our models explainable if, if the model generates something, or if it extracts something, or if it’s trying to make, help you make a decision. We give you citations, we give you references. We make it possible for you to understand how this is happening and and why? Why? The answer is 2.8 where you should go. And so that’s one. The other one is, we make sure that our answers are are grounded in the facts. And there’s, there’s a whole conversation about that. I can I can get deeper into it if you’re interested. But basically what we do is we don’t rely on the intrinsic knowledge of auto regressive models alone. We make sure that they have access to the right tools to go and find information where we trust that information. And then the third step, which is very important, is giving humans full control over what is happening and keeping humans in the loop and enabling them to review what is being generated, what is being extracted, what is being done and when they are part of the process, this part is really important. When they are part of the process in the right way, you are able to deal with a lot of risks that way to make sure that what what you do actually is correct and accurate, and it meets the standards
Madeline Durrett 14:24:56
and as financial institutions also face heightened scrutiny on ESG reporting, is arteria AI developing solutions to streamline ESG compliance. So
Speaker 1 14:25:08
one of the beauties of what we have built at arteria is that this is a system that you can take and you can repurpose it, and you can, we call it fine tuning. So you can take the knowledge system, which is the AI under the hood, and you can further train it, fine tune it for for many different use cases and verticals, and ESG is one of them, and anything that falls under the umbrella of of documentation, and anything that that you can define it in this way that I want to find and access information in different formats and and bring them together and use that information to do something with it, whether you want to use it for reporting, whether you want to do it for making decisions, whatever you want to do, you can you can Do it with our models that we have built, all you need to do is to take it and to configure it to do what you want to do. ESG is one of the examples. And there are lots of other things that you can use our AI for.
Madeline Durrett 14:26:33
And I want to pivot to arterias cafe, because you are the chief science officer at arteria cafe. So the cafe, which is arterias research arm, was launched in January. Could you elaborate on the primary mission of arteria Cafe, and how does it contribute to AI innovation in various use cases such as compliance. Yeah,
Speaker 1 14:26:59
sure, definitely so. When I joined arteria back in four, four and a half years ago, we started building an AI system that would help you find information in the documents. And we built a document understanding solution that is is flexible, it’s fast, it’s accurate, it’s everything that that you want for for document understanding in in the process of doing that, we started discovering new use cases and new things and new ways of doing things that that we we thought there’s a huge opportunity in doing that, but to tame it and to make it work, you would need. Have a focused time, and the right team and the right scientist to be working on that, to de risk it, to figure it out, to make it work. And what we thought was to build art area AI Cafe, which is, as you said, is a is a research arm for art area and and this is where we, we bring real world problems to the to to our lab, and then we bring the state of the art in AI today, and we see there is a gap here. So you need to push it forward. You need to innovate, you need to do research, you need to do whatever you need to do to to use the best AI of today and make it better to be able to solve these problems. That’s what we do in arterial cafe. And our team is a is an interdisciplinary team of of scientists, the best scientists you can find in Canada and in the world. We have brought them here and and we are focused on solving real world problems for for our clients, that’s what we do.
Madeline Durrett 14:29:19
Are there some recent breakthroughs uncovered by arterial cafe or some specific pilot projects in the works you can tell me about?
Speaker 1 14:29:27
You bet. So arterial Cafe is very new. It’s we have been around for a quarter, and usually the answer you get to that question is, it’s too early. Should give us time, and which is true, but because we have been working in this domain for some time, we identified our first thing that we wanted to focus on and and we created something called graph it. Graph it is our innovative way of making generative AI, large language models work flawlessly on on on graph data in a way that is about 10 times less expensive than the the other methods that that were known before and also give You high, highly accurate results when you want to do inference on graphs. And where do you use graphs? You use graphs for AML anti money laundering and a lot of compliance applications. You use it to predict further steps in a lot of actions that you want to take and and there are lots of use cases for these graph analysis that we are using. And with this, we are able to apply and solve problems where you don’t have a lot of training data, as you know, training data, gathering training data, high quality training data, is expensive, it is slow, and in a lot of cases, especially in compliance, suddenly you have you have new regulation, and you have to solve the problem as fast as possible in an accurate way graph. It is an interesting approach that allows us to do all of that without a lot of training data, with minimal training data, and in an inexpensive way and really accurate.
Madeline Durrett 14:31:51
So is this still in the developmental phase, or are you planning on rolling it out soon? We
Speaker 1 14:31:57
actually, we wrote a paper on that, and we submitted it to the web conference 2025, we are going to present it in the web conference in Sydney in about two weeks. That’s
Madeline Durrett 14:32:15
exciting. It’s very exciting. So in addition to your own research arm, how do you collaborate with banks regulators and fintechs to explore new applications of AI and financial services?
Speaker 1 14:32:30
So our approach is this, you, you focus on figuring out new things that that you can do, which are, which are very new. And then you see you can do 15 things, but it doesn’t mean that you should do 15 things. Because life is short and and you need to pick your priorities, and you need to decide what you want to do. So what we do is we work closely with our clients to test what we have, and to do rapid iterations and and to work with them to see, to get feedback on on 15 things that we could focus our efforts on, and, and that is really valuable information to help us decide which direction to take and, and what is it that actually will solve a bigger problem for the work today,
Madeline Durrett 14:33:37
you and we’ve been hearing more talk about agentic AI lately. So what are some use cases for agentic AI and financial services that you see gaining traction and the next three to five years? Next
Speaker 1 14:33:50
three to five years. So what I think we are all going to see is a new type of of software that will be created and and this new type of software is very useful and interesting and very flexible, in the sense that with the traditional software building, even AI software building, you have one goal for your system, and and your system does one thing with the agentic approach and and Using compound AI systems, that is going to change. And you’re going to see software that you build it initially for, for some reason, and and this software, because it is powered by, by this massive sources of of reasoning, llms, for example, this is going to be able to generalize to use cases that you might not have initially thought of, and it will enable you to solve more complex problems more more easily and and that generalization aspect of it is going to be huge, because now you’re not going to have a one trick pony. You will have a system that receives the requirements of what you want to do, and depending on what you want to do. It uses the right tool, uses the right data and and it pivot into the right direction to solve the problem that you want to solve. And with that, you can imagine that to be useful in in many different ways. For example, you can have agentic systems that would work for you, to figure to connect to the outside world and find and collect data for you, and help you make decisions and help you take steps in the direction that you want. For example, you want to apply somewhere for something you don’t have to do it yourself. You can have agents who are that are assistance for you and and they will help you do that. And also, on the other side, if you’re if you’re a bank, you can imagine these agentic systems helping you deal with all of these knowledge intensive tasks that you have at hand and and they help you deal with all the the mess that we have to deal with when we when we work with so much data
Madeline Durrett 14:36:50
that’s pretty groundbreaking. So what else is in the pipeline for arteria AI that you could tell me about.
Speaker 1 14:36:58
So over the past few months, we have built and we have built some very first versions of the next generation of the tools and systems that can solve problems for our clients. In the coming months, we are going to be focused on converting these into applications that we can start testing with our clients, and we can start showing game, showing them to the outside world, and we can start getting more feedback, and you will see great things coming out of our area, because our cafe is full of ideas and full of great things that we have built. I’m
Madeline Durrett 14:37:51
really excited. Thank you. Again to arteria cafe, Chief Science Officer, Dr Amir Hahn, you’ve been listening to the buzz a bank automation news podcast. Please follow us on LinkedIn, and as a reminder, you can rate this podcast on your platform of choice. Thank you all for your time, and be sure to visit us at Bank automation news.com for more automation. News,
14:38:19
thank you. Applause.